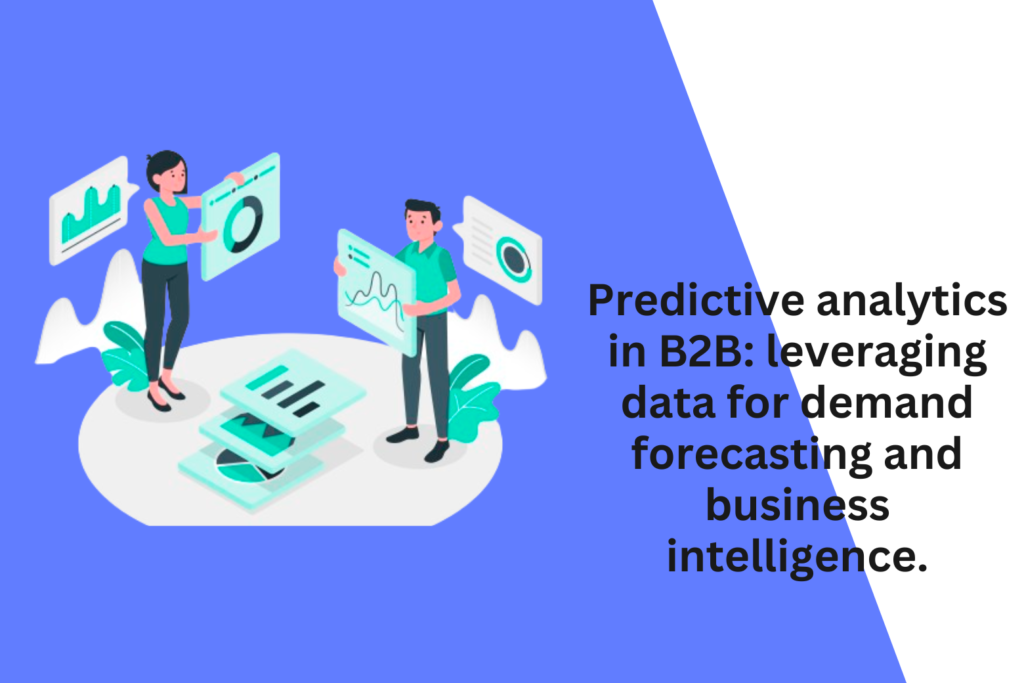
Predictive analytics in B2B involves using advanced statistical algorithms and machine learning techniques to analyze historical data and make predictions about future trends and outcomes. In the context of demand forecasting and business intelligence, here’s how organizations can leverage predictive analytics for strategic insights:
1. Demand Forecasting:
Historical Data Analysis:
- Opportunity: Utilize historical sales data, customer behavior patterns, and market trends to identify patterns and correlations that can inform demand forecasts.
- Benefit: Improved accuracy in predicting future demand, enabling more effective inventory management and production planning.
Customer Segmentation:
- Opportunity: Apply predictive analytics to segment customers based on buying behavior, preferences, and potential lifetime value, enabling targeted marketing and personalized offerings.
- Benefit: Tailoring strategies to different customer segments, optimizing resource allocation and improving customer satisfaction.
Seasonal and Trend Analysis:
- Opportunity: Identify and analyze seasonal patterns and trends in historical data to anticipate fluctuations in demand during specific periods.
- Benefit: Proactively adjust marketing strategies, inventory levels, and staffing to align with seasonal variations.
External Factors Integration:
- Opportunity: Integrate external factors such as economic indicators, industry trends, and geopolitical events into predictive models to enhance the accuracy of demand forecasts.
- Benefit: A more comprehensive understanding of the factors influencing demand, allowing for proactive planning and risk mitigation.
2. Business Intelligence:
Customer Retention Analysis:
- Opportunity: Predictive analytics can help identify indicators of customer churn by analyzing historical data, allowing organizations to implement proactive retention strategies.
- Benefit: Improved customer retention rates and a deeper understanding of factors impacting customer loyalty.
Cross-Selling and Upselling Opportunities:
- Opportunity: Analyze customer purchase history and behavior to predict opportunities for cross-selling and upselling additional products or services.
- Benefit: Increased revenue and enhanced customer relationships through targeted and relevant product recommendations.
Risk Management and Fraud Detection:
- Opportunity: Use predictive analytics to assess and predict potential risks, including identifying fraudulent activities, ensuring compliance, and managing credit risks.
- Benefit: Proactive risk mitigation, improved decision-making, and enhanced security measures.
Operational Efficiency Optimization:
- Opportunity: Predictive analytics can optimize various aspects of operations, including supply chain management, resource allocation, and production schedules.
- Benefit: Improved efficiency, cost reduction, and better resource utilization based on data-driven insights.
3. Lead Scoring and Sales Optimization:
Lead Prioritization:
- Opportunity: Implement predictive lead scoring models to prioritize leads based on their likelihood to convert, allowing sales teams to focus on high-potential prospects.
- Benefit: Increased sales efficiency, improved conversion rates, and better alignment between marketing and sales efforts.
Sales Forecasting:
- Opportunity: Predictive analytics can assist in forecasting future sales by analyzing historical sales data, market trends, and other relevant factors.
- Benefit: Improved accuracy in sales projections, enabling organizations to set realistic targets and allocate resources effectively.
4. Implementation Best Practices:
Data Quality and Integration:
- Best Practice: Ensure the quality and integrity of data by regularly auditing and cleaning datasets. Integrate data from various sources for a holistic view.
- Rationale: Reliable predictions depend on the accuracy and completeness of the data used in predictive models.
Continuous Model Validation and Optimization:
- Best Practice: Regularly validate and optimize predictive models to ensure they remain accurate and relevant as business conditions evolve.
- Rationale: Markets and customer behaviors change, and predictive models need to adapt to these changes to maintain effectiveness.
Cross-Functional Collaboration:
- Best Practice: Foster collaboration between data scientists, business analysts, and domain experts to develop predictive models that align with business goals.
- Rationale: Combining technical expertise with business knowledge ensures that predictive analytics delivers actionable insights.
Scalability and Accessibility:
- Best Practice: Design predictive analytics solutions that are scalable and accessible across different departments and user levels within the organization.
- Rationale: Scalable solutions accommodate growing datasets and user needs, while accessibility promotes widespread adoption.
Conclusion:
Predictive analytics in B2B provides organizations with powerful tools for demand forecasting and business intelligence. By leveraging historical data, customer insights, and external factors, organizations can make informed decisions, optimize operations, and stay ahead in a dynamic business environment. Implementing best practices and maintaining a focus on data quality and adaptability are essential for maximizing the benefits of predictive analytics in B2B settings.